2. ChatGPT and Generative AI in Science
ChatGPT and large language models (LLMs) have been all the rage for nearly a year now and are still producing exciting surprises. Enthought has been active in the discussion with the broader scientific community, where we have looked at this with a scientific and practical lens (see webinar What Every R&D Leader Needs to Know About ChatGPT and LLMs). That same tempered and practical view was echoed at the conference.
As we discussed ChatGPT with other scientists, it was generally acknowledged that the use cases for ChatGPT in science are still very niche but also quite powerful. For example, as we’ve seen in our own work, text summarization does help accelerate preliminary research but is not a game changer on its own. It's going to take a while to explore all the scientific use cases for ChatGPT.
While ChatGPT did not appear in many talks I attended, as both academia and industry are still figuring out its true potential, Generative AI certainly did. We recently wrote about this in our newsletter. There were several talks showcasing how Generative AI can be used for molecular design, from polymers to pharmaceuticals. I expect this number to grow next year as the research community explores more use cases and defines best practices for them. As we tell our clients, it's just a matter of time before Generative AI becomes a standard tool in the molecular design toolkit.
3. Digital Skills for Scientists and Managers
The number of scientists graduating with digital skills is increasing. This is not unexpected, and there is great demand for this talent. It will however take a while before the supply of talent meets the demand, but there are ways to mitigate that (like internal training). The bigger issue we see is that most organizations are not ready to effectively leverage that talent.
Based on our conversations at the conference, and through our consulting business in general, this lack of readiness is largely an organizational and strategic issue. It takes not only the right skills to leverage AI and ML for science, but strategies for picking the right projects to work on; collaborative models that incorporate business, IT, science, and analytical stakeholders; policies to incentivize participation in digital initiatives; and critically, a desire to go beyond one-size-fits-all solutions to more varied and complex challenges. There is a big difference between ‘doing digital’ and ‘being digital.’
Some of the work presented at the conference this year will prove to be transformative, but few organizations are properly equipped to identify and quickly incorporate these innovative methods into their product development process. As the prevalence of digital and industry-specific topics grows at the conference, the ways in which industry can effectively leverage digital tools will become more evident and eventually seen as imperative as part of a continuous improvement approach. Until then, we're here to help!
4. Open-Source for Scientific Innovation
When you see all of the work presented at the conference, it becomes clear that there is so much more room to innovate in this space. Similar technical topics appeared in multiple sub-industry tracks, but that's how you can tell that a technology is making an impact. That is typically facilitated by the availability of open-source software (OSS) or leads to its creation. Many of us at Enthought cut our teeth in graduate school by creating and sharing open-source software that supported our scientific research.
The same thing is happening at the ACS Fall Meeting. There was an entire track dedicated to OSS this year, and so much evidence in other tracks and poster sessions for the value it brings to the community. I recommend browsing titles and looking for software that is relevant to your work. There's a good chance someone else has at least started on a software tool to help with your current R&D challenge.
5. R&D Data Management Platforms
Data management is a big topic, so I attended the Big Data in Chemistry symposium to learn about the emerging use cases presented there. One of the industrial speakers in that track said something to the effect of, "We're doing science and engineering. We don’t have big data. We have small data." I found this timely, as I recently presented a webinar with C&EN on the subject.
There are as many data challenges as there are solutions. It goes to show how tricky "data management" is in materials science and chemistry specifically. We had many conversations about data-driven solutions while at the conference, and found that there is a growing acknowledgement that a focus on data management alone will not lead to accelerated innovation and better problem solving. Data management is necessary, but not sufficient, for R&D digital transformation to make a real impact. Many are disenchanted towards AI, machine learning, and digitalization when they figure this out after having invested heavily in technology that did not (and could not) meet their expectations.
We said to them what we say to our clients—innovation does not come in a box. To unlock the full potential of your scientific data and your experts, it's imperative to take a holistic approach and look end-to-end: the full product development workflow, the right-fit technologies, and the people using the technology. You don't have to transform the entire workflow at once, but you should start the journey with a vision of where you want to end up. From there you can be very practical about how you want to proceed given realistic budget restrictions, time constraints, and risk aversion. Without this, you will likely end up with an incomplete and disappointing solution that doesn’t actually bring real value to your organization.
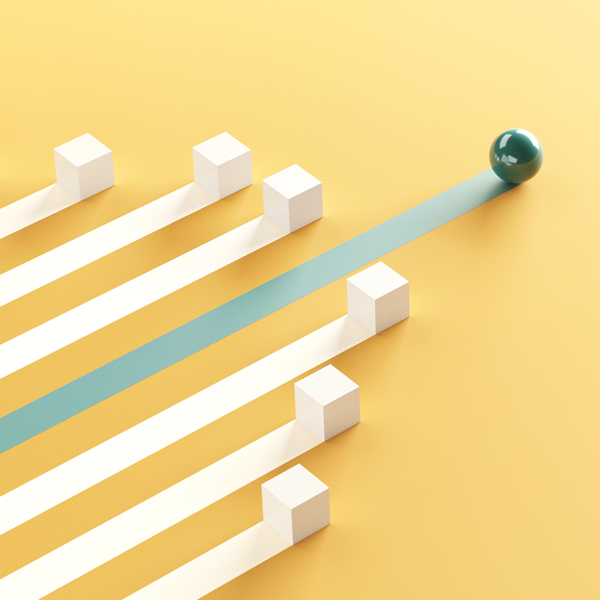
The team left the ACS Fall Meeting energized about the direction of the field and excited about the focus on harnessing scientific data for discovery and product development.
Did you go? Would love to hear your thoughts - connect with me on LinkedIn.
Mike Heiber, Ph.D., Director, Professional Services & Customer Success, Materials Informatics
Related Content
「収益性の壁」を超える:AIの活用で機能性材料開発を戦略から再構築
スペシャルティケミカルおよび素材産業は、コモディティ化と価格競争の激化により、従来の差別化戦略では持続的成長が難しくなっています。こうした中、AIやマテリアルズインフォマティクス(MI)などの先端技術が、R&D戦略の再構築と成長再加速への有力な打ち手として注目されています。
研究開発組織の変革を成功させるためのパートナー選び
現在の競争が激しいR&D環境において、適切なテクノロジーパートナーを選ぶことは、組織にとって最も重要な意思決定の1つです。理想的なパートナーとは、単なるツールベンダーやシステムインテグレーターではなく、生産性を向上させ、イノベーションを加速し、競争力を引き出す解決策を提供する科学的な専門知識と戦略的な洞察を兼ね備えた「変革の同志」です。
「AIスーパー・モデル」が材料研究開発を革新する
近年、計算能力と人工知能の進化により、材料科学や化学の研究・製品開発に変革がもたらされています。エンソートは常に最先端のツールを探求しており、研究開発の新たなステージに引き上げる可能性を持つマテリアルズインフォマティクス(MI)分野での新技術を注視しています。
デジタルトランスフォーメーション vs. デジタルエンハンスメント: 研究開発における技術イニシアティブのフレームワーク
生成AIの登場により、研究開発の方法が革新され、前例のない速さで新しい科学的発見が生まれる時代が到来しました。研究開発におけるデジタル技術の導入は、競争力を向上させることが証明されており、企業が従来のシステムやプロセスに固執することはリスクとなります。デジタルトランスフォーメーションは、科学主導の企業にとってもはや避けられない取り組みです。
産業用の材料と化学研究開発におけるLLMの活用
大規模言語モデル(LLM)は、すべての材料および化学研究開発組織の技術ソリューションセットに含むべき魅力的なツールであり、変革をもたらす可能性を秘めています。
科学研究開発における効率の重要性
今日、新しい発見や技術が生まれるスピードは驚くほど速くなっており、市場での独占期間が大幅に短縮されています。企業は互いに競争するだけでなく、時間との戦いにも直面しており、新しいイノベーションを最初に発見し、特許を取得し、市場に出すためにしのぎを削っています。
R&D イノベーションサミット2024「研究開発におけるAIの大規模活用に向けて – デジタル環境で勝ち残る研究開発組織への変革」開催レポート
去る2024年5月30日に、近年注目のAIの大規模活用をテーマに、エンソート主催のプライベートイベントがミッドタウン日比谷6FのBASE Qで開催されました。
科学研究開発における小規模データの最大活用
多くの伝統的なイノベーション主導の組織では、科学データは特定の短期的な研究質問に答えるために生成され、その後は知的財産を保護するためにアーカイブされます。しかし、将来的にデータを再利用して他の関連する質問に活用することにはあまり注意が払われません。
デジタルトランスフォーメーションの実践
デジタルトランスフォーメーションは、組織のデジタル成熟度を促進し、ビジネス価値を継続的に提供するプロセスです。真にビジネスを変革するためには、イノベーションを通じて新しい可能性を発見し、企業が「デジタルDNA」を育む必要があります。